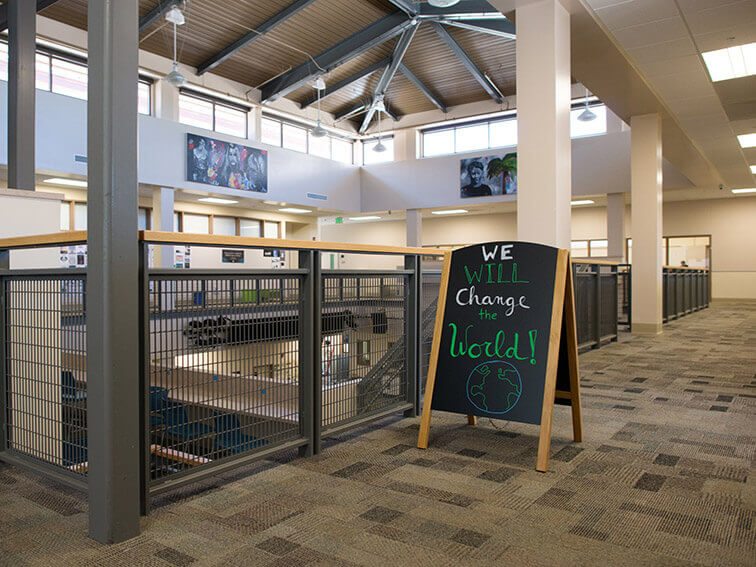
Building MECE Hypotheses Using A Decision Tree
contributed by Ethan Andrews
What is a MECE Hypothesis?
This is quite the mouthful and unless you are a consultant, it likely makes no sense. Simplified, it typically means, to figure out the fundamental root cause of a specific problem, you will need to properly organize and carefully analyze the necessary data, properly consider all the likely possibilities to accurately determine the root cause and make recommendations for the most efficient solution.
Now, while that sounds straightforward enough, when applied to real business problems, the comprehensive approach is most definitely more complex and systematic. To comprehend how the MECE, hypothesis-driven structure and decision tree all align to systematically structure a framework to analyze a key problem, we will begin by looking at each of these specific terms and processes individually.
MECE
As defined in a MECE case interview article by MyConsultingCoach, the MECE rule is one of the fundamental supports of effective communication in consulting. This fundamental rule specifies how key data of the specific case should be exclusively sorted and organized to ensure all possibilities are considered and exhausted. This is a structured, logical way to structure, group and analyze case data.
ME (Mutually Exclusive)
ME (Mutually Exclusive) data can only be organized and grouped into one category. If this specific data can be organized into more than one category, the data is not mutually exclusive. ME data should be exclusive to one category and not overlap.
An example of a framework that is NOT Mutually Exclusive.
The problem you are represented with is a global brand of baby products that are experiencing an issue with a consistent decrease in profits. You have grouped the issue into two categories for analysis.
- Profits in Europe
- Profits for nappies, formulas and lotions
This grouping is not mutually exclusive as both categories will overlap. While analyzing profits in Europe, you will have to analyze these profits across all categories of baby products. This means the comparative analysis is duplicated and not ME (mutually exclusive).
Typically categorizing and grouping specific data by geographical location for analysis would ME:
- Analyze direct profits by the specific geographical market (US/Europe/Africa)
CE (Collectively Exhaustive)
The second specific part of this framework represents the variables of the data to ensure the analysis is collectively exhaustive. This means the comparative analysis is thorough in an efficient way that it properly includes all possible causes of the client’s fundamental problem.
In order to ensure the root cause is identified, you have to ensure that all possibilities contributing to the issue with the profits are considered. Now, while you have grouped the ME data, in order to ensure that all possibilities are considered, you need to expand on the categories.
For example:
- .US
- .Formula
- .500gr tins
- .1kg tins
- .Nappies
- .Small
- .Medium
- .Large
- .Formula
Summary: MECE is a framework that allows you to organize data to ensure that all data is analyzed to ensure all possibilities are exhausted.
What Is A Hypothesis Driven Approach?
This comprehensive approach is remarkably fundamental actually. Once you have correctly identified the possible issues, a hypothesis-driven approach effectively means you will consider each possible cause to determine if this is the root cause of the problem. In fundamental essence, this approach enables you to narrow down the expanses of data you will investigate until you are left with the most likely cause.
So if you have identified A, B & C as the possible problem, the hypothesis-driven approach means that you analyze and consider first each possibility until you are left with the concluding result. This approach goes hand in hand with structuring the problem and is something you will find is covered in detail in case interview preparation.
The Decision Tree
A Decision Tree is a comprehensive framework that will enable you to outline, draft and carefully consider the possible outcomes to each possible course of corrective action to remedy the fundamental problem. This organizational structure will enable you to consider the potential risks and the possible rewards of each possible solution until you can narrow down the most likely solution.
A decision tree is exactly what it sounds like. You will typically start with the tree trunk (the problem) and then include the branches, which represent each of the possible solutions. This is just a general description and is a topic covered in more detail in case interview preparation.
In Summary
To summarize and outline the descriptive term, “How to Build MECE Hypotheses Using a Decision Tree.”
- Start by understanding the fundamental problem. You must ensure you have a clear, precise understanding of what the potential problem is.
- Properly organize and diligently prepare the necessary data into logical MECE groups to define the key issue.
- Develop a problem driven formula and use the hypothesis to narrow down the likely possibilities.
- Using a decision tree, consider the outcomes of each possible solution to remedy the root cause of the problem.